Data-driven saliency models have proved to accurately infer human visual attention on real images. Reusing such pre-trained models in video games holds significant potential to optimize game design and storytelling. However, the relevance of transferring saliency models trained on real static images to dynamic 3D animated scenes with stylized rendering and animated characters remains to be shown. In this work, we address this question by conducting a user study that quantitatively compares visual attention obtained through gaze recording with the prediction of a pre-trained static saliency model in a 3D animated game environment. Our results indicate that the tested model was able to accurately approximate human visual attention in such conditions.
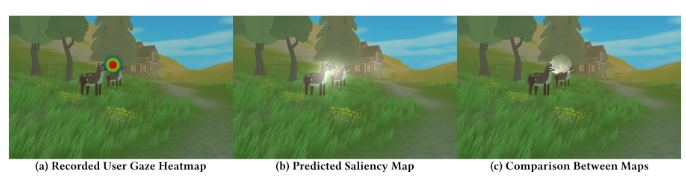
https://project.inria.fr/mig2023/files/2023/11/poster_6.pdf
METHODOLOGY In our user experiment, participants sat in a well-lit room behind a clear background. They faced an RGB camera1 used by the eyetracking software GazeRecorder2 and a monitor screen displaying the virtual world. After calibrating the software to capture landmarks on the subject’s image, we record their gaze data as they navigated the virtual environment through a fixed trajectory which lasted approximately 38 seconds. Once the trajectory was complete, we would stop recording and collect the output provided by GazeRecorder, consisting of two videos: one captures the user’s screen view, while the other presents the same screen recording overlaid with a heatmap representation of the gaze data