This paper presents a novel webcam-based approach for gaze estimation on computer screens. Utilizing appearance based gaze estimation models, the system provides a method for mapping the gaze vector from the user’s perspective onto the computer screen. Notably, it determines the user’s 3D position in front of the screen, using only a 2D webcam without the need for additional markers or equipment. The study presents a comprehensive comparative analysis, assessing the performance of the proposed method against established eye tracking solutions. This includes a direct comparison with the purpose-built Tobii Eye Tracker 5, a high-end hardware solution, and the webcam-based GazeRecorder software. In experiments replicating head movements, especially those imitating yaw rotations, the study brings to light the inherent difficulties associated with tracking such motions using 2D webcams. This research introduces a solution by integrating Structure from Motion (SfM) into the Convolutional Neural Network (CNN) model. The study’s accomplishments include showcasing the potential for accurate screen gaze tracking with a simple webcam, presenting a novel approach for physical distance computation, and proposing compensation for head movements, laying the groundwork for advancements in real-world gaze estimation scenarios.
https://www.frontiersin.org/articles/10.3389/frobt.2024.1369566/full
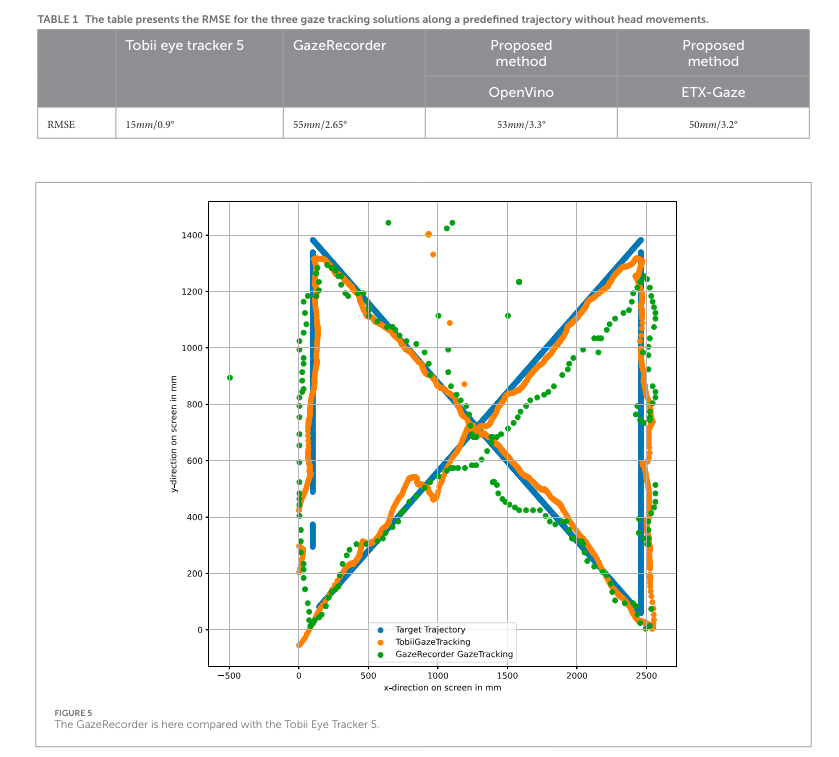
Conclusion and future work
In this study, we presented a novel method for webcam based gaze estimation on a computer screen requiring only four calibration points. Our focus was not on evaluating the precision of any appearance based CNN gaze estimation model but on demonstrating our proposed method on a single user. We presented a methodology to project a gaze vector onto a screen with an unknown distance. The proposed regression model determined a transformation matrix, TGS allowing the conversion of the gaze vector from the gaze coordinate system to the screen coordinate system.
Physical validation of the user’s position in front of the screen confirmed the soundness of our approach, representing a novel method for determining the physical distance and location of a user without stereo imaging. The method’s accuracy is contingent on the precision of the gaze vector model, here the OpenVINO model and a model trained on the ETH-XGaze dataset.
Comparisons with established gaze-tracking solutions, Tobii Eye Tracker 5 and GazeRecorder, showcased comparable results, indicating the potential efficacy of our method. Further experiments addressed head movements, highlighting the limitations of 2D webcam-based solutions and proposing compensation using SfM techniques.
Future work should focus on refining the proposed method to enhance accuracy, especially in uncontrolled environments. Specialized training of CNN models, incorporating user position via SfM, should be explored using data sets like (Zhang et al., 2020 or Tonsen et al., 2016). The CNN trained with these adjustments should be able to effectively differentiate between lateral movements to the left or right and specific head movements, such as yaw and pitch rotations.
Moreover, there is potential for enhancing sensitivity to cope with varying lighting conditions. The introduction of supplementary filtering mechanisms may aid in bolstering accuracy, particularly in challenging lighting scenarios.
Generally, webcam-based gaze tracking solutions, exhibit a notable sensitivity to varying lighting conditions. It is essential to underscore that the overall accuracy of our proposed method is intrinsically tied to the precision of the raw gaze vector generated by the gaze estimation model. In this study we have conclusively demonstrated that webcam-based solutions, while promising, still cannot attain the level of accuracy achieved by sophisticated gaze tracking hardware, which frequently leverages advanced technologies such as stereo vision and infrared cameras. Consequently, the pursuit of further research endeavors is imperative in order to bridge the existing gap and elevate webcam-based solutions to a level of accuracy comparable to that of purpose-built eye tracking hardware.