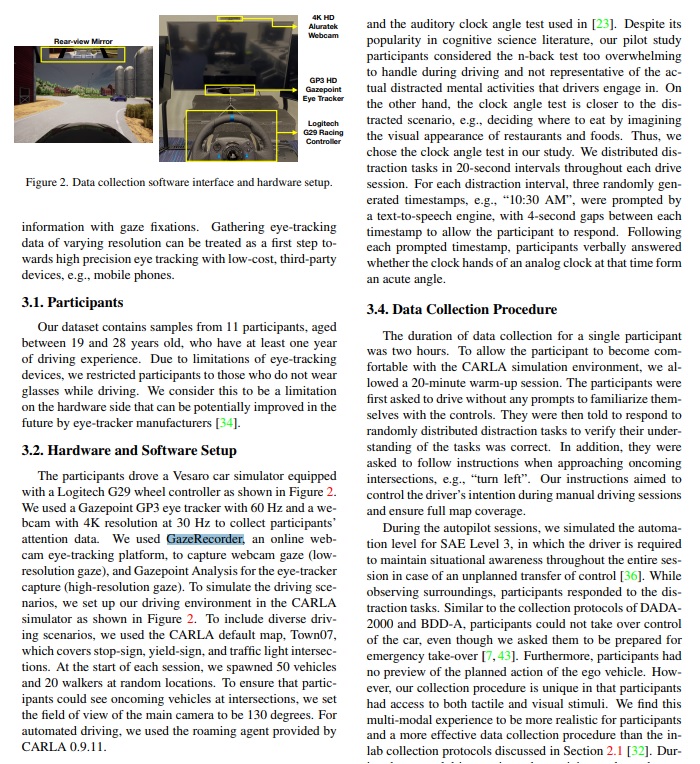
The task of driver attention prediction has drawn considerable interest among researchers in robotics and the autonomous vehicle industry. Driver attention prediction can play an instrumental role in mitigating and preventing highrisk events, like collisions and casualties. However, existing driver attention prediction models neglect the distraction state and intention of the driver, which can significantly influence how they observe their surroundings. To address these issues, we present a new driver attention dataset, CoCAtt (Cognitive-Conditioned Attention). Unlike previous driver attention datasets, CoCAtt includes per-frame annotations that describe the distraction state and intention of the driver. In addition, the attention data in our dataset is captured in both manual and autopilot modes using eyetracking devices of different resolutions. Our results demonstrate that incorporating the above two driver states into
attention modeling can improve the performance of driver attention prediction. To the best of our knowledge, this work is the first to provide autopilot attention data. Furthermore, CoCAtt is currently the largest and the most diverse driver attention dataset in terms of autonomy levels, eye tracker resolutions, and driving scenarios.